The Essential Role of Data Annotation Tools in AI Development
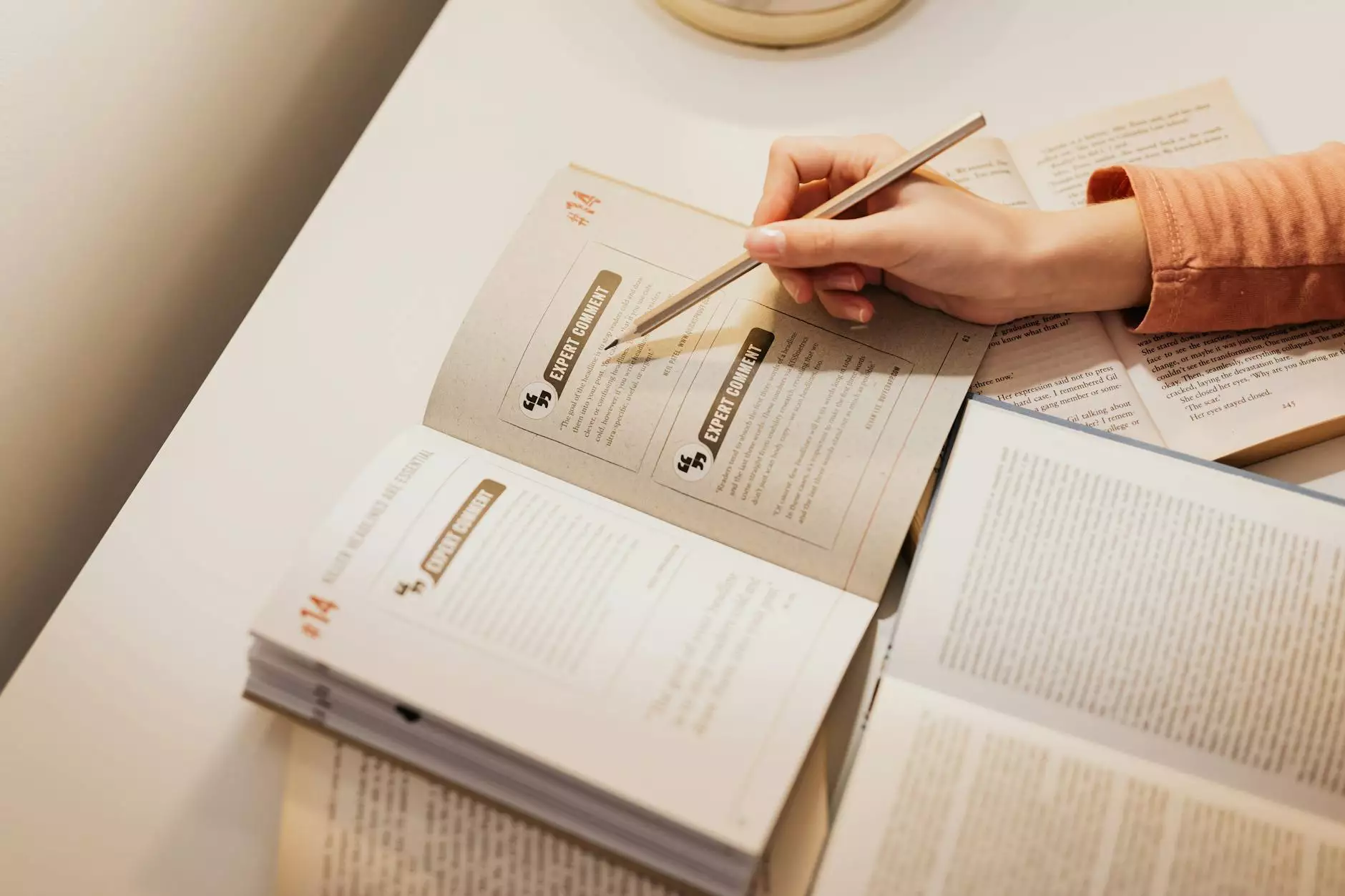
In today's fast-paced digital world, the significance of data annotation tools cannot be overemphasized. As businesses increasingly rely on artificial intelligence (AI) to enhance their operations, the demand for accurately labeled data has surged. These tools facilitate the process of annotating vast datasets, ensuring that algorithms produce accurate and reliable outcomes.
What is a Data Annotation Tool?
A data annotation tool is software designed to assist in the labeling of data, making it suitable for machine learning models. They provide a user-friendly interface for annotators to tag various types of data, including text, images, and audio. These tools are instrumental in preparing datasets for AI algorithms, particularly in domains such as natural language processing (NLP), computer vision, and more.
Types of Data Annotation
Data annotation encompasses various types of tasks, each serving a unique purpose in AI training:
- Image Annotation: Tagging images to help computer vision algorithms recognize objects, faces, and environments.
- Text Annotation: Enhancing textual data with labels that help machines understand sentiments, intents, and entities.
- Audio Annotation: Labeling audio clips to allow voice recognition systems to comprehend spoken language accurately.
- Video Annotation: Annotating video frames for applications in surveillance, autonomous vehicles, and entertainment.
Why Choose a High-Quality Data Annotation Tool?
Investing in a top-tier data annotation tool offers numerous advantages, including:
- Increased Efficiency: High-quality tools streamline the annotation process, reducing the time required to label data accurately.
- Improved Accuracy: Advanced features and AI-assisted functionalities minimize human error, ensuring your labeled data is reliable.
- Scalability: A reputable tool can handle large datasets, making it easier for businesses to expand their AI initiatives.
- Collaboration: Many tools include collaborative features that allow teams to work together seamlessly, regardless of location.
- Adaptability: Versatile tools can cater to various industries, from healthcare to finance to retail.
Features to Look for in a Data Annotation Tool
Selecting the right data annotation platform requires careful consideration of several features:
User-Friendly Interface
The best tools boast an intuitive interface that simplifies the workflow for annotators, enabling them to focus on accurately labeling data.
Support for Multiple Data Types
With various types of data to annotate, a robust tool should support text, images, audio, and video formats.
Quality Assurance Mechanisms
Look for features that help maintain quality control, such as review systems and automated checks to catch potential errors.
Integration Capabilities
A data annotation tool should easily integrate with existing data management systems and machine learning pipelines to ensure a smooth workflow.
Collaboration Features
As teams often work remotely, collaboration features such as shared projects and live commenting can significantly enhance productivity.
Case Study: KeyLabs.ai's Data Annotation Platform
Among the leading providers of data annotation tools, KeyLabs.ai has emerged as a frontrunner in offering comprehensive solutions that cater to diverse business needs. Their platform integrates state-of-the-art technology with a deep understanding of the annotation process.
Key Features of KeyLabs.ai
- AI-Assisted Annotation: KeyLabs.ai uses machine learning techniques to enhance the efficiency and accuracy of the annotation process, allowing human annotators to work faster while maintaining high quality.
- Real-Time Collaboration: Teams can collaborate in real-time, enabling seamless communication and enhancing project outcomes.
- Robust Quality Control: KeyLabs.ai employs stringent quality assurance protocols to guarantee that all annotated data meets rigorous industry standards.
- Flexible Pricing Models: Understanding that each business has unique needs, KeyLabs.ai offers flexible pricing options tailored to various project scopes.
The Importance of Quality Data Annotation
Quality data annotation is critical because poorly labeled data can lead to inaccurate predictions and results in AI applications. A well-annotated dataset enables machine learning algorithms to learn effectively, leading to:
- Enhanced Model Performance: Accurate annotations result in better-performing models capable of delivering reliable outputs.
- Reduced Error Rates: High-quality training data significantly reduces the likelihood of errors in real-world applications.
- Increased Trust in AI: When organizations rely on algorithms for decision-making, the accuracy and reliability of these models build trust within teams and stakeholders.
Best Practices for Effective Data Annotation
To maximize the effectiveness of your data annotation tool, consider implementing the following best practices:
Define Clear Guidelines
Establishing comprehensive guidelines helps annotators understand what is expected, ensuring consistency throughout the dataset.
Use Sample Annotations
Providing sample annotations can serve as a useful reference, enabling annotators to grasp the desired outcomes quickly.
Regular Training Sessions
Investing time in training sessions empowers annotators, enhancing their skills and understanding of the nuances of tagging data correctly.
Implement a Review Process
Incorporating a review process allows for the assessment of annotations, providing learning opportunities for annotators and improving overall data quality.
Future Trends in Data Annotation
The field of data annotation is evolving, and several trends are emerging that are set to shape its future:
AI-Driven Automation
As AI technology advances, we can expect to see more automation integrated into the data annotation process, significantly reducing the time and effort required while improving accuracy.
Increased Demand for Annotated Data
With the rapid growth of AI applications, the need for high-quality annotated data will only continue to rise, making it essential for businesses to invest in reliable tools.
Focus on Ethics and Privacy
As concerns around data privacy grow, ethical data annotation will become paramount, prompting companies to adopt best practices and transparent processes.
Conclusion
In conclusion, data annotation tools are an indispensable part of the AI development process. Choosing the right tool is crucial for businesses that wish to harness the power of AI effectively. Companies like KeyLabs.ai provide excellent solutions that combine technology with user-centered design, offering the flexibility and accuracy needed for today's data-driven world.
With the ongoing advancements in technology and the increasing importance of data in decision-making, investing in high-quality data annotation becomes not just advantageous but essential for any business aiming to thrive in the AI era.